Implementing RCM with Querri
Reliability-centered Maintenance (RCM) enables organizations to optimize maintenance programs, ensuring tasks are done efficiently and cost-effectively while optimizing the reliability of maintained equipment.
An RCM framework can guide the effective use of Predictive Maintenance (PdM). Combined, these approaches to maintenance can achieve truly world-class reliability efficiently.Our focus here is not on these techniques themselves but on how Querri can assist in their implementation.
Implementing these approaches requires significant data collection, cleaning, and analysis. The collection process is often very manual, resulting in a messy data set. Querri can take this messy data, clean it up, automate much of the process of creating an RCM framework, and develop PdM models.
For our examples, we are using a relatively simple dataset designed for examination of Failure Modes with Explainable AI (IEEE - Explainable AI, Kaggle - XAI Drilling Dataset)
Laying the Foundation: Failure Mode and Effects Analysis (FMEA)
RCM uses a Failure Mode and Effects Analysis (FMEA) to understand the components of a system, potential failure modes, causes of those modes, and local, system, and plant level impact of those failures. Querri's Natural Language Processing (NLP) and data processing capabilities can ingest raw data, correct for variances in input wording, and automatically build out the analysis as far as the data allows.

The data set we’re starting with has limited information. With data for Failure Cause, Local, System, Plant, LTA, these could also be auto-populated. In this case, I also populated the Failure Cause column by looking at relationships of other variables. By automating much of this labor-intensive process, Querri enables organizations to more quickly establish a solid foundation for their RCM program, saving valuable time and resources.
Identifying Failure Modes, Effects, and Criticality (FMEA)
Building upon the FMEA, Querri can further support the RCM process using its data exploration and visualization tools to uncover patterns and relationships between operating conditions, failure modes, and their effects.
The exploratory data analysis plots and correlation heat map below provide insights into these relationships, facilitating the identification of critical failure modes and their potential consequences.
When the data is available, Querri can use machine learning techniques to determine optimal scheduling for time-directed or condition-based maintenance activities.
Often, just asking for Querri to do “EDA” will give a lot of good insights, but in this case I specifically asked for the charts.
“Show a pair plot of the data in blue with a KDE diagonal.”

“Show a Heat map of the data.”
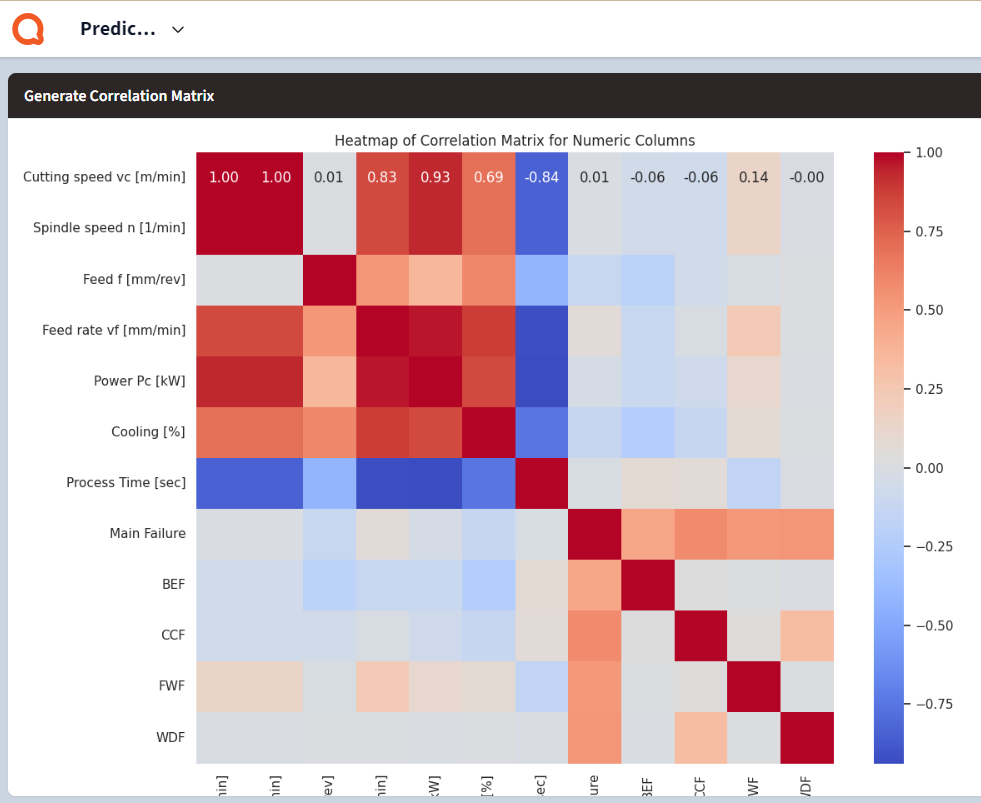
Optimizing Maintenance Strategies with Predictive Modeling
Querri can also help identify the best times to act for Preventative Maintenance, particularly for Time-Directed and Condition-Directed activities. By loading in time and condition data related to prior failures, Querri can build models to predict the optimal times to act to prevent future failures.
Querri can also examine element failures and look for the most important causes of failure, improving forecasting potential failures and their modes based on historical data. When the data is available, Querri can use machine learning techniques to determine optimal scheduling for time-directed or condition-based maintenance activities. As shown in the graph below, the feature importance analysis highlights the most significant predictors for each failure mode, enabling organizations to prioritize and target their maintenance efforts accordingly.
“Show the feature importance for each Failure Type graphically. Put the features in the legend and Failure Types on the Axis.”

By leveraging these predictive insights, organizations can proactively address potential failures, minimize downtime, and optimize maintenance strategies, aligning with the RCM principle of applying the most cost-effective maintenance approach for each scenario.
Continuous Improvement and Optimization
RCM is an iterative process, requiring continuous monitoring, analysis, and refinement of maintenance strategies. Querri's adaptive modeling capabilities support this ongoing improvement cycle by ingesting new data, updating predictions, and providing ever-more accurate insights into asset performance and failure modes. This feedback loop enables organizations to continuously refine their RCM program, driving operational excellence and maximizing asset reliability.

References:
RCM: Gateway to World Class Maintenance by Anthony M. Smith, Glenn R. Hinchcliffe
Predictive Maintenance in the RCM World