Implementing RCM with Querri
Implementing Reliability Centered Maintenance requires significant data collection, cleaning, and analysis. The collection process is often very manual, resulting in a messy data set. Read our related blog here.
Querri can:
- ✓ Take this messy data, clean it up
- ✓ Automate the process of creating an RCM framework
- ✓ And develop PdM models
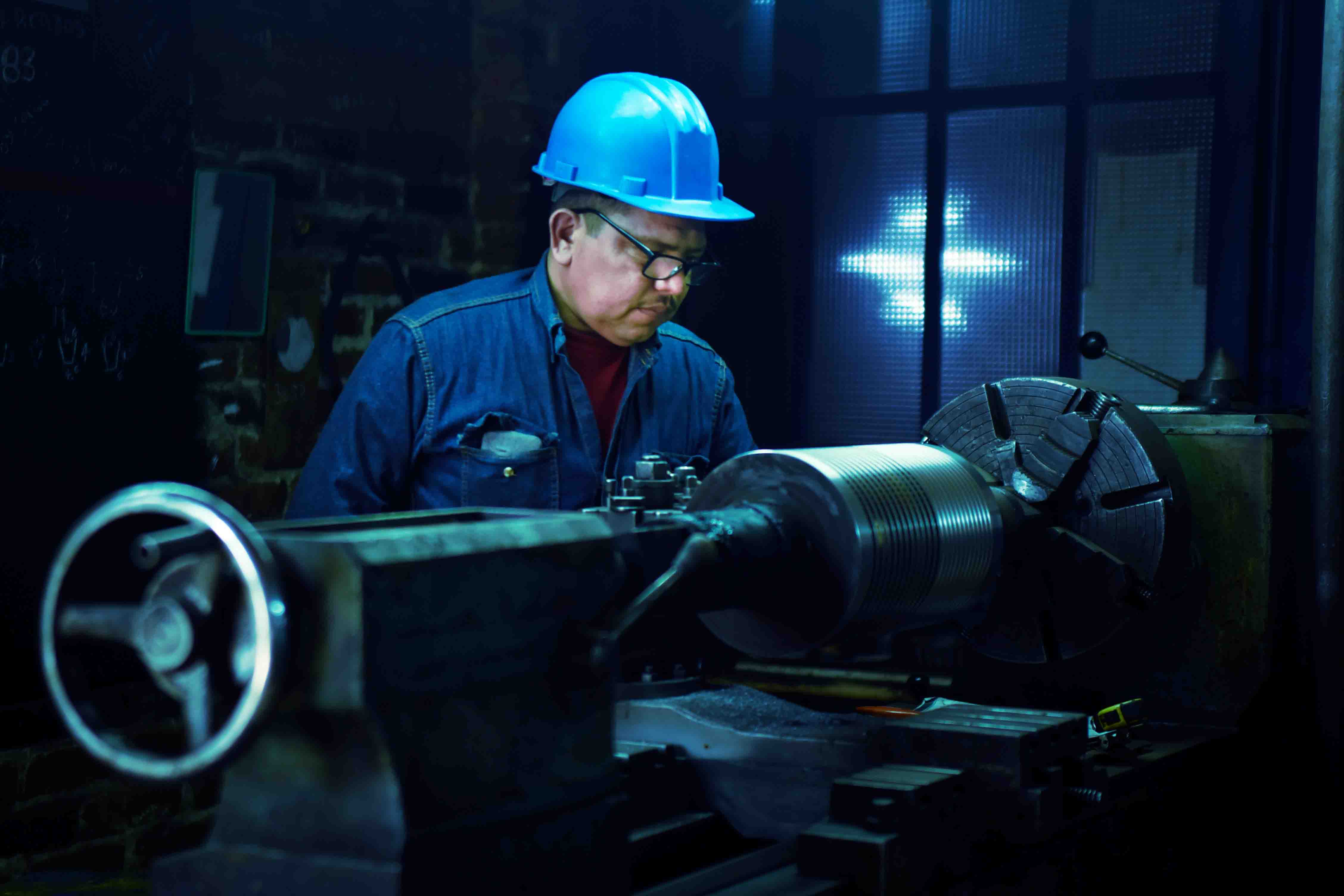
Failure Mode and Effects Analysis (FMEA)
Querri's Natural Language Process and data processing capabilities can help to clean up human-entered data, such as closing comments and filling in missing data in intelligent ways.
This makes it easier to analyze an asset's past failures and potential failure modes, causes of those modes, and their component, asset, and plant-level impact.
You can also read our blog "Supercharging Six Sigma with Querri" here.
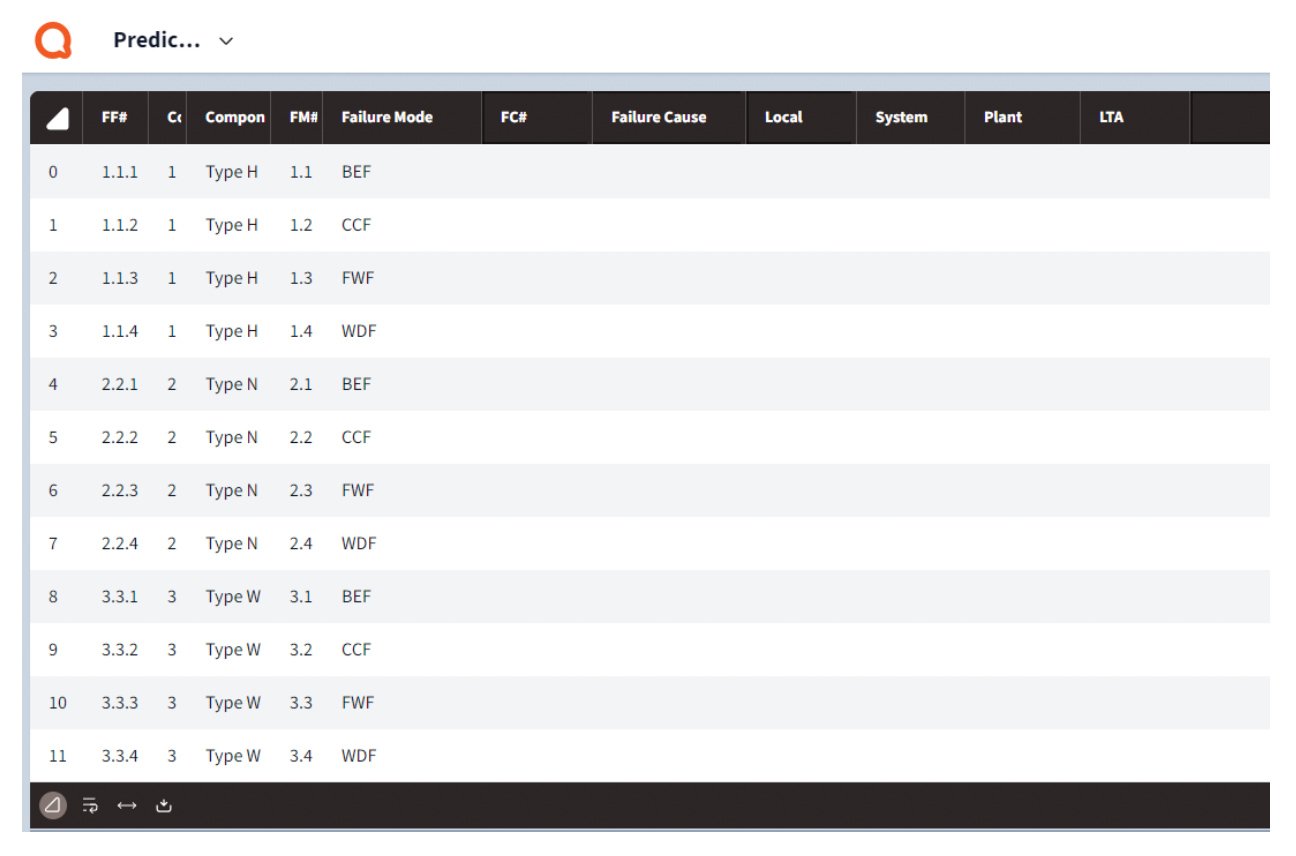
Optimizing Maintenance Strategies with Predictive Modeling
It uses machine learning techniques to determine optimal scheduling for time-directed or condition-based maintenance activities.
Querri can also examine element failures and look for the most important causes, improving forecasting potential failures and their modes based on historical data.
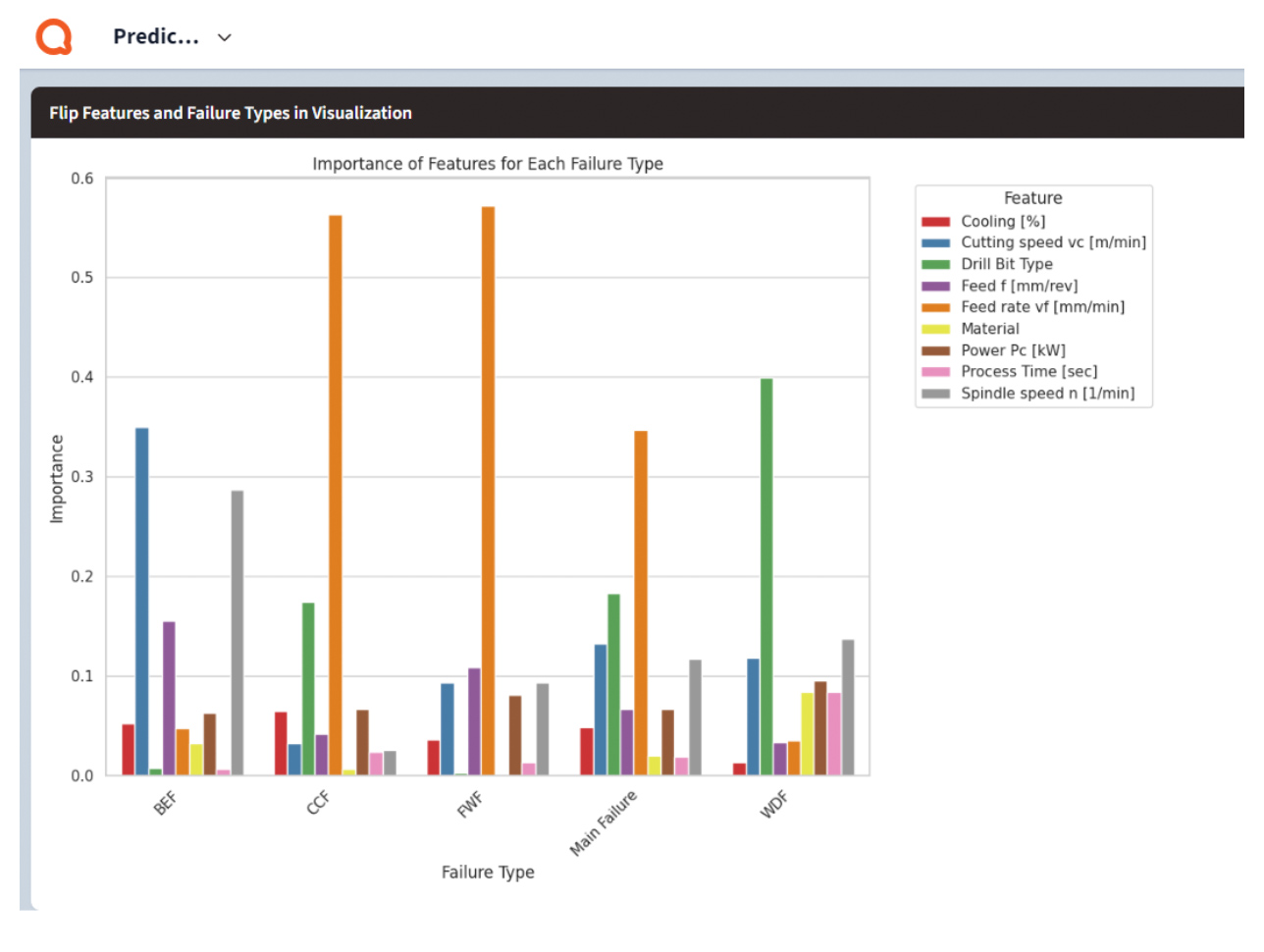